Enhance Your Knowledge with www.informaticsview.com: Insightful Data and Analytics Strategies
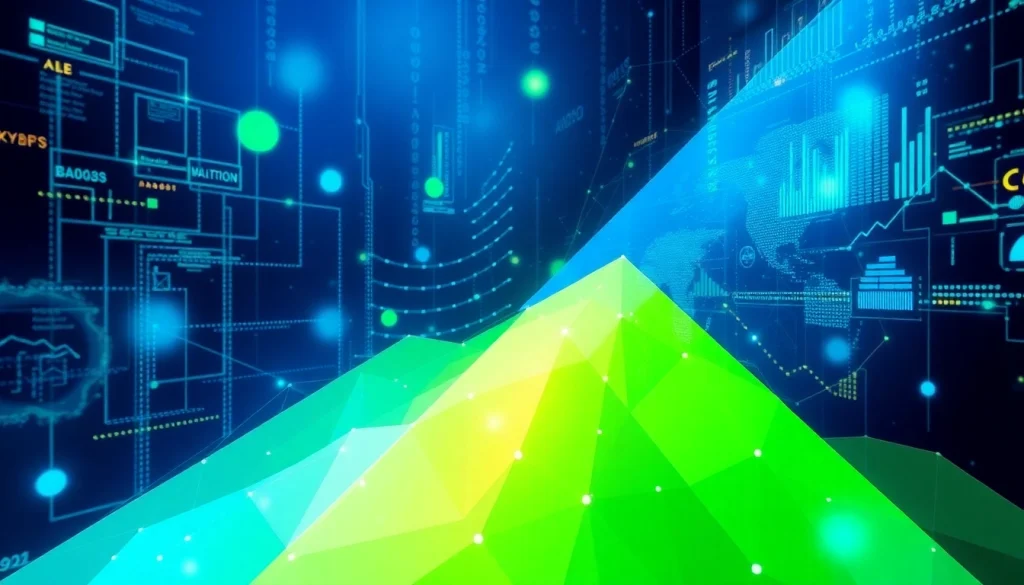
Understanding the Role of Data in Modern Technology
In today’s fast-paced digital landscape, data has emerged as one of the most valuable assets for organizations across industries. The ability to collect, analyze, and leverage data effectively is crucial for staying competitive. Platforms like www.informaticsview.com provide insights and strategies that help businesses navigate this data-driven era. This article delves deep into the role of data in modern technology, exploring its significance, key trends, effective strategies, tools, and real-world applications.
The Importance of Data Visualization
Data visualization is the graphical representation of information and data. By using visual elements like charts, graphs, and maps, complex data sets are transformed into understandable visual formats, making it easier for stakeholders to recognize patterns, trends, and outliers.
Visualization is not just beneficial but essential in today’s data-rich environments. It allows organizations to communicate insights clearly and efficiently. For instance, businesses can illustrate their sales performance by region, helping teams identify where efforts should be concentrated. Furthermore, effective data visualization helps in persuasive storytelling — aligning facts with visual context to influence decision-making.
Types of Data Analytics Explained
Data analytics encompasses a variety of methods and disciplines. Broadly, it can be categorized into four types:
- Descriptive Analytics: This type focuses on summarizing past data to understand trends and patterns. For example, businesses might look at previous sales data to assess performance.
- Diagnostic Analytics: Here, the goal is to understand why something happened by identifying correlations between variables. Companies analyze customer feedback alongside sales data to uncover potential issues that led to a decline in sales.
- Predictive Analytics: This analytic form uses statistical models and machine learning techniques to forecast future outcomes based on historical data. For instance, retailers might predict seasonal buying trends.
- Prescriptive Analytics: This type suggests actions to benefit from the predictions. For example, airlines may use prescriptive analytics to optimize flight schedules based on projected demand.
Understanding these different types helps organizations tailor their analytics strategies to meet specific business goals effectively.
Data-Driven Decision Making Best Practices
Data-driven decision-making (DDDM) leverages data analysis to guide business strategies and practices. Implementing DDDM effectively involves several best practices:
- Define Clear Objectives: Understand what you want to achieve through data analysis, whether it’s increasing sales, improving customer satisfaction, or optimizing operations.
- Encourage a Data Culture: Foster an environment where data is valued across all organizational levels. This encourages employees to base their decisions on data rather than gut feelings.
- Invest in Training: Equip your team with the skills necessary for data analysis, interpretation, and visualization to ensure that everyone can engage effectively with the data.
- Utilize the Right Tools: Employ technologies suited to your organization’s needs, which can streamline data collection and analysis processes.
- Evaluate and Iterate: Continuously assess the impact of data-driven decisions and refine methodologies to enhance effectiveness.
Key Trends in Data Science and Analytics
The field of data science and analytics is continually evolving. Staying updated on emerging trends is crucial for leveraging data effectively. Below are several key trends shaping the landscape.
Emerging Technologies Shaping Analytics
Technological advancements are at the forefront of transforming data analytics. Key emerging technologies include:
- Artificial Intelligence (AI) and Machine Learning: These technologies automate data processing and increase accuracy in predictions. Companies are using AI to enhance customer experiences with personalized recommendations.
- Big Data Technologies: The conventional data storage and processing techniques struggle with the volume, variety, and velocity of big data. Technologies like Hadoop and Spark enable organizations to leverage vast amounts of data for insights.
- Cloud-Based Analytics: Cloud computing solutions provide scalable and flexible analytics tools. Businesses can access real-time data analytics without investing in extensive on-premises hardware.
- Blockchain: While primarily recognized for cryptocurrencies, blockchain offers exceptional data security, transparency, and integrity, making it a valuable asset for industries reliant on data accountability.
Industry Applications of Data Science
Data science has permeated various industries, transforming how businesses operate. Here are a few notable applications:
- Healthcare: Predictive analytics improves patient outcomes by identifying potential health risks before they escalate.
- Finance: Financial institutions use data analytics to detect fraud patterns and assess credit risks, ensuring informed lending decisions.
- Retail: Retailers harness consumer data to optimize inventory, personalize marketing efforts, and enhance customer satisfaction.
- Manufacturing: Data analytics streamlines supply chains by predicting equipment failures and optimizing maintenance schedules.
Future Trends to Watch
As technology continues to advance, several future trends in data science and analytics are emerging:
- Edge Computing: Processing data closer to its source reduces latency and improves response times, which is essential for IoT applications.
- Automated Data Analytics: Automating reporting and analysis tasks will minimize human error and free up analysts to focus on strategic initiatives.
- Enhanced Data Privacy Measures: With growing concerns over data privacy, regulations and technologies ensuring data protection will become increasingly important.
- Integration of Quantum Computing: While still in its infancy, quantum computing holds the potential to process data at incomprehensibly fast rates, revolutionizing analytics capabilities in areas like drug discovery and cryptography.
Implementing Effective Data Strategies
Transitioning to a data-driven organization requires an organized approach to implementing effective data strategies. Below are critical steps to consider.
Steps to Build Your Analytics Model
Creating a robust analytics model involves several steps:
- Identify Business Objectives: Clearly define what you want your analytics model to achieve, aligning it with overall business goals.
- Collect Relevant Data: Gather data from diversified sources to ensure a comprehensive analysis. Sources may include transaction histories, customer interactions, and social media.
- Data Cleaning and Preparation: Raw data often contains errors or inconsistencies. Preparing your data involves cleaning and structuring it so that it’s usable for analysis.
- Choose Analytical Methods: Depending on your objectives, select appropriate analytical techniques – statistical analysis, machine learning models, etc.
- Deploy and Monitor: Implement the analytics model into your business processes and continuously monitor its performance against defined objectives.
Common Challenges and Solutions
While implementing data strategies, organizations may encounter various challenges:
- Data Quality Issues: Poor data quality can lead to misleading insights. To counter this, develop strict data governance policies and invest in data quality tools.
- Resistance to Change: Employees may be reluctant to adopt new data-driven processes. Initiating training sessions and demonstrating the value of data insights can help ease this transition.
- Resource Limitations: Some organizations lack the necessary talent or technology resources. Consider partnering with data consultancy services or investing in relevant training programs for existing staff.
Measuring Success: KPIs and Metrics
Data strategy success is gauged through Key Performance Indicators (KPIs) tailored to specific business goals. Common metrics to assess include:
- Customer Acquisition Cost (CAC): Evaluates the resources invested in acquiring a new customer. Monitoring CAC can help refine marketing strategies.
- Return on Investment (ROI): Calculating the ROI from data initiatives reveals their financial effectiveness.
- Customer Lifetime Value (CLV): This metric measures the total revenue a business can expect from a customer over their entire relationship, providing insights into customer value.
- Data Accuracy: Monitoring data accuracy helps maintain trust in analytical outputs and drives more informed business decisions.
Tools and Technologies for Data Analysis
The right tools can significantly enhance data analysis capabilities. Here’s a breakdown of popular technologies and considerations when choosing the right stack.
Comparing Popular Data Analytics Tools
Data analytics tools vary greatly, catering to different organizational needs. Below is an overview of popular options:
- Tableau: Renowned for its powerful data visualization capabilities, Tableau enables users to create interactive, shareable dashboards and is user-friendly.
- Power BI: Microsoft’s tool integrates seamlessly within the Microsoft ecosystem, offering robust analytics and visualization functionalities.
- Python and R: Both programming languages excel in data analysis and are pivotal for building custom models due to their flexibility and strong community support.
- SAS: This software suite offers advanced analytics, business intelligence, and data management capabilities, making it a popular choice for enterprises.
The Role of AI in Data Processing
Artificial Intelligence is revolutionizing data processing by introducing automation and advanced analytical capabilities. AI can facilitate:
- Automated Insights: By utilizing machine learning algorithms, AI can identify trends in real-time, allowing companies to act swiftly on emerging data insights.
- Predictive Analytics: AI models can synthesize vast amounts of data to offer predictions and recommendations, transforming how businesses approach planning and strategy.
- Natural Language Processing (NLP): NLP enables machines to understand and interpret human language, improving user interfaces for data analytics tools.
Choosing the Right Technology Stack
Selecting an appropriate technology stack involves considering several factors:
- Business Needs: Clarify the specific needs of your organization; different tools serve varied purposes — visualization, predictive analytics, or data integration.
- Scalability: Choose tools that can grow with your business, ensuring that they can handle increasing data volumes without sacrificing performance.
- User Experience: Select user-friendly tools that team members can easily learn and use to foster adoption and effective usage.
- Cost: Factor in the total cost of ownership, including software licenses, infrastructure, and training expenses to determine your budget.
Case Studies: Success Stories in Data Utilization
Exploring real-world examples of data utilization can provide valuable insights into effective strategies and common pitfalls.
Industry Leaders Leveraging Data Effectively
Numerous organizations have successfully leveraged data for competitive advantage:
- Amazon: Through extensive data analytics, Amazon personalizes recommendations for customers, enhancing user experience and driving sales.
- Netflix: Utilizing viewer data, Netflix effectively curates content for specific demographics, leading to increased customer engagement and satisfaction.
- Walmart: By analyzing its vast data sets, Walmart optimizes inventory and supply chain processes, leading to improved efficiency and cost savings.
Lessons Learned from Data Failures
Not all data initiatives meet success. Analyzing failures provides critical insights into potential pitfalls:
- Target’s Data Breach: In 2013, Target suffered a significant data breach, highlighting the importance of robust cybersecurity measures in data management.
- Healthcare.gov: The launch of the Healthcare.gov website faced severe data integration issues, underscoring the need for thorough testing and planning prior to implementation.
Strategies for Continuous Improvement
Continuous improvement is key to maintaining data strategy effectiveness. Strategies include:
- Regular Feedback Loops: Establish mechanisms for employees to provide feedback on data initiatives, facilitating ongoing refinement of processes.
- KPI Adjustments: Regularly review and adjust KPIs to align them with evolving business goals, ensuring that they remain relevant and impactful.
- Investing in Training: As new tools and techniques evolve, continuous education for employees is essential to keep the organization’s data capabilities sharp.
- Staying Informed on Trends: Regularly engaging with current research in data science and analytics will help organizations stay ahead of trends and technology updates.