Revolutionizing Information Retrieval: The Future of AI Search Technology
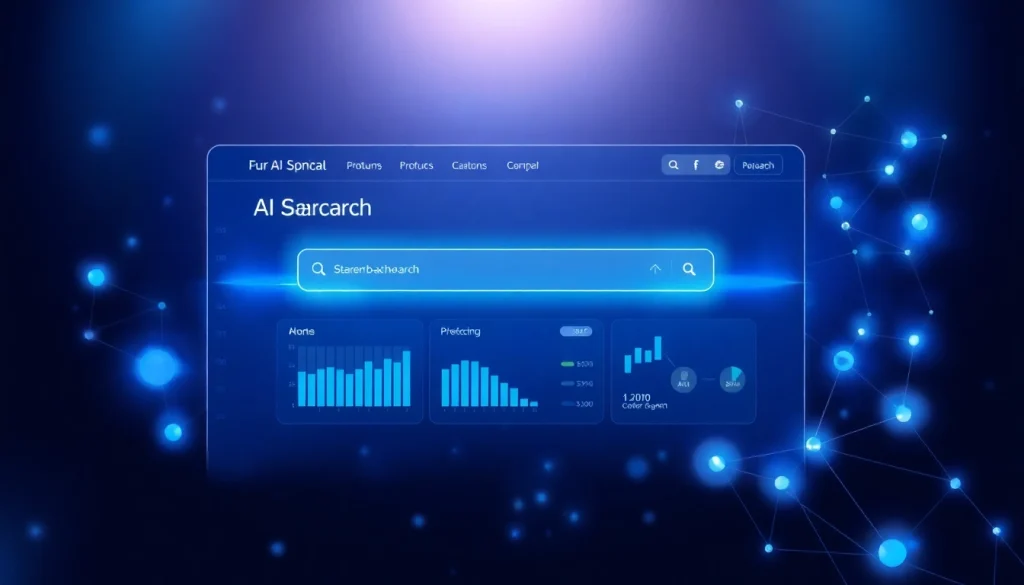
Understanding AI Search: Fundamentals and Innovations
As the digital landscape evolves, the way we search for information must also adapt. Traditional search engines have served us well for decades, but the introduction of AI search technologies heralds a new era of information retrieval that promises to transform our interactions with data. This article explores the foundations of AI search, the innovative technologies that drive it, the benefits it offers, the challenges it poses, and the future it holds.
What is AI Search?
AI search refers to the use of artificial intelligence technologies to enhance and optimize the process of searching for information. While traditional search engines use keyword matching and indexing to return results, AI search leverages machine learning, natural language processing (NLP), and advanced algorithms to understand user intent, grasp the context of search queries, and provide more relevant and personalized results. This sophisticated approach aims to not only deliver information but also to anticipate the needs of users and facilitate deeper understanding.
Key Technologies Behind AI Search
The effectiveness of AI search engines is fueled by a variety of key technologies, each contributing to their capability to comprehend and deliver information efficiently:
- Natural Language Processing (NLP): NLP enables search engines to interpret and process human language, allowing them to understand context, nuances, and intent behind queries.
- Machine Learning: This technology allows search engines to continually learn from user interactions and improve over time, leading to more accurate and efficient search results.
- Semantic Search: By understanding the meaning behind words and phrases, semantic search helps deliver results based on the context rather than just keyword matches, thus improving relevance.
- Data Analytics: AI search employs analytics to monitor user behavior, engagement metrics, and feedback, which inform ongoing improvements and personalization.
- Multimodal Search: This technology supports searching across various data formats, such as images, text, and voice, enabling richer user experiences and facilitating comprehensive information retrieval.
Benefits of Implementing AI Search Solutions
Organizations that adopt AI search solutions experience a multitude of benefits, which include:
- Enhanced User Experience: AI search engines provide faster, more relevant results, improving user satisfaction and engagement.
- Increased Efficiency: By accurately predicting user needs and minimizing the rank of irrelevant results, AI search reduces the time users spend searching.
- Better Insights: Data collected from user interactions with AI search systems helps organizations understand how users find and consume information, paving the way for informed decision-making.
- Personalization: Tailored search results, based on individual user preferences and behaviors, create a more engaging experience.
Types of AI Searches Available
Comparing Various AI Search Engines
The market is saturated with various AI search engines, each offering distinct features and capabilities. Some notable ones are:
- Google AI Search: Harnessing the power of its advancements in NLP and machine learning, Google continuously refines its search technology, integrating multimodal capabilities with tools like Google Lens.
- Microsoft Azure AI Search: Aimed at enterprises, this solution combines AI with knowledge retrieval systems, making it particularly useful for businesses with complex data needs.
- ChatGPT Search: Developed by OpenAI, this model enhances traditional search with conversational prompts, leading to more informative and efficient interactions with search data.
- Felo AI: As a multilingual answer engine, Felo allows users to ask questions across various languages and receive instant answers, emphasizing accessibility.
Enterprise vs. Consumer AI Searches
AI search solutions can be broadly categorized into enterprise and consumer versions. Enterprise solutions generally exhibit more robust features to handle large datasets and complex queries, making them suitable for organizational contexts. In contrast, consumer AI searches focus on simplicity, user engagement, and accessibility, catering primarily to individual users.
Understanding Multimodal AI Search
Multimodal AI search represents a paradigm shift in how searches can be conducted. Rather than restricting users to textual inputs, multimodal search enables interactions through different formats such as text, voice, images, and videos. For instance, by combining visual search queries with text-based inputs, users can retrieve results that are both contextually and semantically relevant. This approach not only improves search accuracy but also enriches the user experience by accommodating diverse interaction methods.
Challenges in AI Search Implementation
Addressing Citation and Content Accuracy Issues
One of the persistent challenges facing AI search engines is ensuring the accuracy and verifiability of information. Traditional sources of reliability, such as citations and bibliography, often get overlooked in the context of AI-generated content. As highlighted in reports about AI search engines, many fail to consistently provide proper citations, leading to misinformation. To counter this issue, developers must prioritize embedding source verification mechanisms and employing sophisticated algorithms that assess information quality before displaying it to users.
Handling User Data Privacy Concerns
With a growing emphasis on data privacy and security, AI search technologies must navigate complex regulatory environments. Implementing AI search solutions often involves processing large volumes of user data, raising valid concerns regarding privacy breaches and unauthorized use of personal information. To build trust, organizations should be transparent about data collection practices, adhere to legal frameworks like GDPR, and employ strong encryption methods to protect user data.
Navigating the AI Search Landscape Effectively
The AI search landscape is rapidly evolving, creating a diverse ecosystem where businesses must continually adapt to changes. Staying ahead of competitors requires insight into emerging trends and collaboration between organizations, researchers, and regulatory bodies. Companies should invest in research and development to refine their algorithms, engage in partnerships for shared knowledge, and monitor developments in related fields such as algorithmic fairness and bias reduction.
Best Practices for AI Search Optimization
Key Metrics to Measure AI Search Success
To evaluate the performance of AI search systems, organizations should track various key metrics, including:
- Precision and Recall: These metrics assess the relevance of search results, indicating how well the system can deliver pertinent information.
- User Engagement: Tracking metrics such as click-through rates and time spent on pages can provide insights into user satisfaction and search effectiveness.
- Conversion Rates: Measuring how many users perform desired actions after engaging with search results helps assess overall success.
- Error Rates: Monitoring the frequency of incorrect results can highlight areas for improvement in the AI search algorithms.
Ensuring Seamless User Experience
A seamless user experience is essential for maximizing the potential of AI search. This can be achieved by minimizing load times, simplifying interfaces, and creating intuitive navigation paths. Additionally, providing personalized recommendations based on user behavior and preferences enhances engagement and keeps users coming back.
Continuous Improvement Strategies for AI Searches
To maintain competitive advantage, businesses need to embrace continuous improvement in their AI search capabilities. This can be facilitated through:
- Regular Updates: Continuous refinement of algorithms and features keeps search functionalities up to date with user expectations and technological advancements.
- User Feedback: Actively seeking user engagement in the form of feedback can identify pain points and provide direct insight into areas needing enhancement.
- Cross-functional Teams: Collaboration across technical experts, UX designers, and marketing teams ensures holistic improvements that meet user needs and business objectives.
The Future of AI Search Technologies
Predictions for AI Search Advancements
The future of AI search is poised to witness transformative advancements, driven by the convergence of technologies. Predictions suggest increasingly personalized search experiences, improved understanding of user intents, and the advent of more interactive interfaces. The integration of voice-assisted technology may also redefine the nature of searches, making them more conversational and user-friendly.
Impact of Quantum Computing on AI Search
Quantum computing has the potential to revolutionize AI search architectures by dramatically increasing processing power. This can facilitate complex calculations at previously unimaginable speeds, taking AI search capabilities to the next level. Organizations that embrace quantum technologies will be able to process vast amounts of data in real-time, leading to instantaneous queries and supremely accurate results.
How to Adapt to Emerging Trends in AI Search
To thrive in the rapidly evolving world of AI search, companies should be proactive in adapting to emerging trends. This includes investing in research and development, forming strategic partnerships to leverage new technologies, and remaining agile to pivot based on user feedback and shifting market demands. Organizations must cultivate a culture of innovation and agility, ensuring they remain at the forefront of changes in AI search methodologies and user expectations.